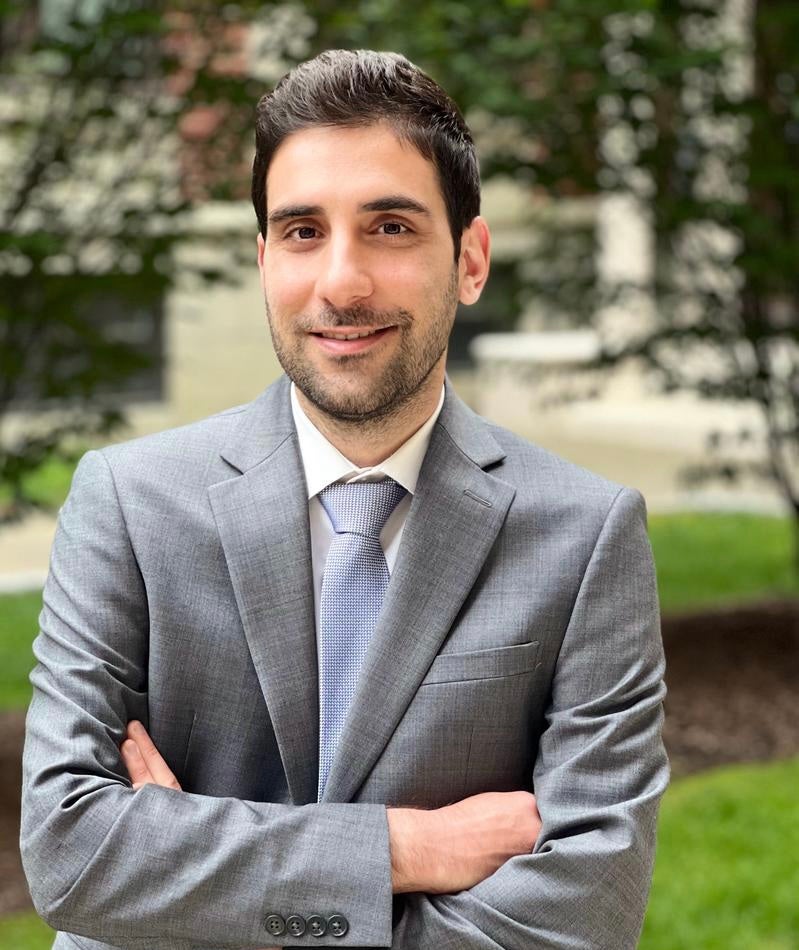
About
Dr. Amiri was appointed an Assistant Professor in the Department of Computer Science at RPI in Fall 2023. His research revolves around the theme of collective intelligence. In today's rapidly evolving technological landscape, harnessing the power of data at the edge of the network has emerged as a pivotal catalyst for unlocking the true potential of collective intelligence. As the world becomes increasingly interconnected, the wealth of information generated at the periphery of our networks holds the key to a new era of innovation and insight. By tapping into this decentralized data wealth, we not only empower individuals and devices with real-time decision-making capabilities but also foster a collaborative environment where a multitude of perspectives converge to form a unified intelligence. This collective intelligence transcends traditional boundaries, enabling us to tackle complex challenges with unprecedented efficiency and creativity. Embracing the untapped potential of data at the edge is not just a technological advancement; it's a strategic imperative that drives us towards a future where our connected world thrives on the synergy of decentralized insights, propelling us to reach heights we never thought possible. Dr. Amiri's research focuses on using this decentralized data to enhance the system intelligence beneficial for everyone while protecting the sensitive information. Please visit his homepage for more information about his research.
Dr. Amiri received the Ph.D. degree in Electrical and Electronic Engineering form Imperial College London in 2019. He further received the M.Sc. degree in Electrical and Computer Engineering from the University of Tehran in 2014, and the B.Sc. degree in Electrical Engineering from the Iran University of Science and Technology in 2011, both with the highest rank. He is the recipient of the Best Ph.D. Thesis award from both the Department of Electrical and Electronic Engineering at Imperial College London during the academic year 2018-2019, as well as the IEEE Information Theory Chapter of UK and Ireland in the year 2019. Also, his paper titled “Federated learning over wireless fading channels” received the IEEE Communications Society Young Author Best Paper Award in the year 2022.
During his academic experience, Dr. Amiri held two Postdoctoral Associate appointments, one in the Department of Electrical and Computer Engineering at Princeton University and one in the Media Lab at Massachusetts Institute of Technology. These prestigious academic havens provided him with an invaluable opportunity to collaborate with pioneering minds and delve into cutting-edge research. These formative experiences not only honed his technical prowess but also instilled in him a passion for imparting knowledge and fostering the next generation of innovative thinkers.
Prospective graduate students: I am looking for motivated Ph.D. students with strong mathematical and analytical skills. Please send me your CV if you are interested in Machine Learning and comfortable with programming.
Ph.D. Electrical and Electronic Engineering, Imperial College London, 2019 (Best Ph.D. Thesis)
M.Sc. Electrical and Computer Engineering, University of Tehran, 2014 (Highest Honors)
B.Sc. Electrical Engineering, Iran University of Science and Technology, 2011 (Highest Honors)
Research
My research interests revolve around the theme of enabling collective intelligence. Consider the continuously increasing number of connected devices with the emergence of the Internet-of-Things paradigm and various smart sectors generating a significant amount of data. Tailoring machine learning algorithms to exploit this massive amount of data can lead to many new applications and open-up new markets in medical care, finance, and enabling ambient intelligence. Due to the privacy concerns and the growing storage and computational capabilities of edge devices, it is increasingly attractive to store and process the data locally by shifting network computations to the edge. This enables decentralized intelligence where local computations on the data converts decentralized data to a global intelligence; hence, enhancing data privacy while learning from the collection of data. My research focuses on creation of a collective intelligence using all the data that is inherently decentralized and only visible to its owner. Please visit my homepage to learn more about my research.
Teaching
- CSCI 4960\6960: Machine Learning Seminar
Recognition
IEEE Communications Society Young Author Best Paper Award (2022), paper "Federated learning over wireless fading channels", IEEE Transactions on Wireless Communications, vol. 19, no. 5, pp. 3546-3557, May 2020.
Best PhD Thesis (2019), IEEE Information Theory Chapter of UK and Ireland.
Eryl Cadwallader Davies Prize - Outstanding PhD Thesis (2019), EEE Department, Imperial College London.
EEE Departmental Scholarship (2015 - 2019), Imperial College London.
Excellent Student (2014), Ranked 1st among all M.Sc. students in ECE Department, University of Tehran.
Excellent Student (2011), Ranked 1st among all B.Sc. students in EE Department, Iran University of Science and Technology.
Outstanding Student Award (2007 - 2011), Iran University of Science and Technology.
"Collective intelligence", Bell Labs, May 2023.
"Decentralized data valuation", Decentralized Society + Web3 Research Panel, Media Lab Fall Meeting, Massachusetts Institute of Technology, Oct. 2022.
"Federated edge machine learning", Keynote speaker at Futurewei University Days Workshop, Aug. 2021.
"Federated edge learning: advances and challenges", Keynote speaker at IEEE International Conference on Communications, Networks and Satellite (COMNETSAT), Dec. 2020.
"Federated edge learning: advances and challenges", King's College London, Nov. 2020.
"Federated edge learning: advances and challenges", University of Maryland, Oct. 2020.
"Federated edge learning: advances and challenges", University of Arizona, Oct. 2020.
"Federated learning: advances and challenges", Virginia Polytechnic Institute and State University (Virginia Tech), Oct. 2020.
"Fundamental limits of coded caching", Ohio State University, Nov. 2016.
"Fundamental limits of coded caching", Stanford University, Nov. 2016.
Publications
Book Chapters
M. Mohammadi Amiri and D. Gunduz, Machine learning and wireless communications, (edited by H. Vincent Poor, Yonina Eldar, Andrea Goldsmith, and Deniz Gunduz), Cambridge University Press, Cambridge, UK, 2021.
M. Mohammadi Amiri and D. Gunduz, Edge caching for mobile networks, (edited by H. Vincent Poor and Wei Chen), IET Press, London, UK, 2021.
Journal Papers
Y.-S. Jeon, M. Mohammadi Amiri, and N. Lee, Communication-efficient federated learning over MIMO multiple access channels, IEEE Transactions on Communications, vol. 70, no. 10, pp. 6547-6562, Oct. 2022.
H. Hellstrom, J. M. da Silva, M. Mohammadi Amiri, M. Chen, V. Fodor, H. V. Poor, and C. Fischione, Wireless for machine learning: a survey, Foundations and Trends in Signal Processing, vol. 15, no. 4, pp. 290-399, Jun. 2022.
M. Mohammadi Amiri, D. Gunduz, S. R. Kulkarni, and H. V. Poor, Convergence of federated learning over a noisy downlink, IEEE Transactions on Wireless Communications, vol. 21, no. 3, pp. 1422-1437, Mar. 2022.
M. Mohammadi Amiri, T. M. Duman, D. Gunduz, S. R. Kulkarni, and H. V. Poor, Blind federated edge learning, IEEE Transactions on Wireless Communications, vol. 20, no. 8, pp. 5129-5143, Aug. 2021.
M. Mohammadi Amiri, D. Gunduz, S. R. Kulkarni, and H. V. Poor, Convergence of update aware device scheduling for federated learning at the wireless edge, IEEE Transactions on Wireless Communications, vol. 20, no. 6, pp. 3643-3658, Jun. 2021.
Y.-S. Jeon, M. Mohammadi Amiri, J. Li, and H. V. Poor, A compressive sensing approach for federated learning over massive MIMO communication systems, IEEE Transactions on Wireless Communications, vol. 20, no. 3, pp. 1990-2004, Mar. 2021.
D. Gunduz, D. Burth Kurka, M. Jankowski, M. Mohammadi Amiri, E. Ozfatura, and S. Sreekumar, Communicate to learn at the edge, IEEE Communication Magazine, vol. 58, no. 12, pp. 14-19, Dec. 2020.
J. Zhao, M. Mohammadi Amiri, and D. Gunduz, Multi-antenna coded content delivery with caching: a low-complexity solution, IEEE Transactions on Wireless Communications, vol. 19, no. 11, pp. 7484-7497, Nov. 2020.
M. Mohammadi Amiri and D. Gunduz, Federated learning over wireless fading channels, IEEE Transactions on Wireless Communications, vol. 19, no. 5, pp. 3546-3557, May 2020 (IEEE Communications Society Young Author Best Paper Award).
M. Mohammadi Amiri and D. Gunduz, Machine learning at the wireless edge: distributed stochastic gradient descent over-the-air, IEEE Transactions on Signal Processing, vol. 68, pp. 2155-2169, Apr. 2020.
M. Mohammadi Amiri and D. Gunduz, Computation scheduling for distributed machine learning with straggling workers, IEEE Transactions on Signal Processing, vol. 67, no. 24, pp. 6270-6284, Dec. 2019.
Q. Yang, M. Mohammadi Amiri, and D. Gunduz, Audience-retention-rate-aware caching and coded video delivery with asynchronous demands, IEEE Transactions on Communications, vol. 67, no. 10, pp. 7088-7102, Oct. 2019.
M. Mohammadi Amiri and D. Gunduz, Caching and coded delivery over Gaussian broadcast channels for energy efficiency, IEEE Journal on Selected Areas in Communications, vol. 36, no. 8, pp. 1706-1720, Aug. 2018.
M. Mohammadi Amiri and D. Gunduz, Cache-aided content delivery over erasure broadcast channels, IEEE Transactions on Communications, vol. 66, no. 1, pp. 370-381, Jan. 2018.
M. Mohammadi Amiri, Q. Yang, and D. Gunduz, Decentralized caching and coded delivery with distinct cache capacities, IEEE Transactions on Communications, vol. 65, no. 11, pp. 4657-4669, Nov. 2017.
M. Mohammadi Amiri and D. Gunduz, Fundamental limits of coded caching: Improved delivery rate-cache capacity trade-off, IEEE Transactions on Communications, vol. 65, no. 2, pp. 806-815, Feb. 2017.
M. Mohammadi Amiri and B. Maham, Two novel adaptive transmission schemes in a decode-and-forward relaying network, Wireless Personal Communications, vol. 96, no. 4, pp. 5705-5722, Oct. 2017.
M. Mohammadi Amiri, A. Olfat, and N. C. Beaulieu, Novel beamforming scheme for multicasting in cooperative wireless networks with a multiple antenna relay, IEEE Transactions on Wireless Communications, vol. 14, no. 8, pp. 4482-4493, Aug. 2015.
Conference Proceedings
Z. Wu, M. Mohammadi Amiri, R. Raskar, and B. K. H. Low, Incentive-aware federated learning with training-time model rewards, under review.
P. Vapakomma, M. Mohammadi Amiri, C. L. Canonne, R. Raskar, and A. Pentland, Private independence testing across two parties, under review.
A. Chopra, S. K. Sahu, A. Singh, A. Java, P. Vepakomma, M. Mohammadi Amiri, and R. Raskar, Adaptive split learning, Conference on Machine Learning and Systems (MLSys), Miami Beach, FL, USA, Jun. 2023.
K. Yang*, M. Mohammadi Amiri*, and S. Kulkarni, Greedy centroid initialization for federated K-means, Conference on Information Sciences and Systems, Baltimore, MD, USA, Mar. 2023.
* Equal contribution.M. Mohammadi Amiri, F. Berdoz, and R. Raskar, Fundamentals of task-agnostic data valuation, AAAI Conference on Artificial Intelligence, Washington DC, USA, Feb. 2023.
M. Mohammadi Amiri, S. R. Kulkarni, and H. V. Poor, Federated learning with downlink device selection, IEEE International Workshop on Signal Processing Advances in Wireless Communications (SPAWC), Lucca, Italy, Sep. 2021.
M. Mohammadi Amiri, D. Gunduz, S. R. Kulkarni, and H. V. Poor, Federated learning with quantized global model updates, submitted for publication, Jun. 2020.
M. Mohammadi Amiri, D. Gunduz, S. R. Kulkarni, and H. V. Poor, Update aware device scheduling for federated learning at the wireless edge, IEEE International Symposium on Information Theory (ISIT), Los Angeles, CA, USA, Jun. 2020.
M. Mohammadi Amiri, T. M. Duman, and D. Gunduz, Collaborative machine learning at the wireless edge with blind transmitters, IEEE Global Conference on Signal and Information Processing (GlobalSIP), Ottawa, Canada, Nov. 2019.
M. Mohammadi Amiri and D. Gunduz, Machine learning at the wireless edge: distributed stochastic gradient descent over-the-air, IEEE International Symposium on Information Theory (ISIT), Paris, France, Jul. 2019.
M. Mohammadi Amiri and D. Gunduz, Over-the-air machine learning at the wireless edge, IEEE International Workshop on Signal Processing Advances in Wireless Communications (SPAWC), Cannes, France, Jul. 2019.
J. Zhao, M. Mohammadi Amiri, and D. Gunduz, A low-complexity cache-aided multi-antenna content delivery scheme, IEEE International Workshop on Signal Processing Advances in Wireless Communications (SPAWC), Cannes, France, Jul. 2019.
M. Mohammadi Amiri and D. Gunduz, Computation scheduling for distributed machine learning with straggling workers, IEEE International Conference on Acoustics, Speech, and Signal Processing (ICASSP), Brighton, UK, 2019.
M. Mohammadi Amiri and D. Gunduz, On the capacity region of a cache-aided Gaussian broadcast channel with multi-layer messages, IEEE International Symposium on Information Theory (ISIT), Colorado, USA, Jun. 2018.
M. Mohammadi Amiri and D. Gunduz, Decentralized caching and coded delivery over Gaussian broadcast channels, IEEE International Symposium on Information Theory (ISIT), Aachen, Germany, Jun. 2017.
Q. Yang, M. Mohammadi Amiri, and D. Gunduz, Audience retention rate aware coded video caching, IEEE International Conference on Communications (ICC), Paris, France, May 2017.
M. Mohammadi Amiri and D. Gunduz, Cache-aided data delivery over erasure broadcast channels, IEEE International Conference on Communications (ICC), Paris, France, May 2017.
M. Mohammadi Amiri, Q. Yang, and D. Gunduz, Decentralized coded caching with distinct cache capacities, Asilomar Conf. on Signals, Systems and Computers, Pacific Grove, CA, Nov. 2016.
M. Mohammadi Amiri and D. Gunduz, Improved delivery rate-cache capacity trade-off for centralized coded caching, IEEE International Symposium on Information Theory and Its Applications (ISITA), Monterey, CA, Oct.-Nov. 2016.
M. Mohammadi Amiri, Q. Yang, and D. Gunduz, Coded caching for a large number of users, IEEE Information Theory Workshop (ITW), Cambridge, UK, pp. 171-175, Sep. 2016.
M. Mohammadi Amiri and A. Olfat, Low complexity adaptive transmission scheme for cooperative networks with decode-and-forward relay, International Symposium on Telecommunications (IST), Tehran, Iran, pp. 1128-1132, Sep. 2014.
The following is a selection of recent publications in Scopus. Mohammad Mohammadi Amiri has 32 indexed publications in the subjects of Computer Science, Engineering, Mathematics.
Openings
I am looking for motivated Ph.D. students with strong mathematical and analytical skills. Please send me your CV if you are interested in Machine Learning and comfortable with programming.