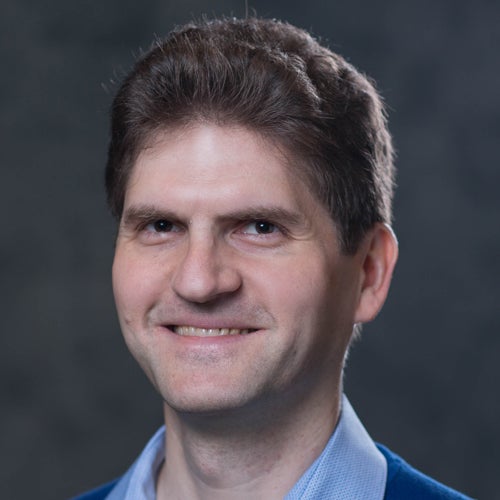
-
331 Amos Eaton Hall
- kramep@rpi.edu
-
518-276-6896
- 0000-0003-4867-5835
About
Dr. Kramer received his B.A. in Physics from Princeton University, and earned his Ph.D. in Applied and Computational Mathematics at Princeton University under the supervision of Professor A.J. Majda. Dr. Kramer took a three-year Courant Instructor and NSF postdoctoral research fellow position at the Courant Institute at New York University, before joining the faculty in the Department of Mathematical Sciences at Rensselaer as an assistant professor in 2000.
Dr. Kramer's research focuses on the application of ideas and techniques from probability theory and differential equations to model and analyze complex systems which evolve in time and involve too many variables to represent explicitly in a practical computational model. The effects of the unresolved variables on the quantities of interest are treated in a statistical fashion. Current areas of research include transport within biological cells, the swimming dynamics of irregular colonies of flagellated cells, statistical modeling in environmental science, and the statistical inference of neuronal network topology from firing data. This work is in collaboration with scientists and engineers at Arizona State University, University of Colorado at Boulder, as well as at Rensselaer.
Dr. Kramer supervises the preparation of undergraduates at Rensselaer for the annual Mathematical and Interdisciplinary Contest in Modeling, and supports the organization of the Mathematical Problems in Industry Workshop (2000-present).
Ph.D., Princeton University, 1997
Research
I apply ideas from probability theory and differential equations to model and analyze complex systems which evolve in time and involve too many variables to represent explicitly in a practical computational model. The effects of the unresolved variables on the quantities of interest are treated in a statistical fashion. My current applications of interest are:
- understanding how diverse molecular motor proteins facilitate transport in cells and shape the spindle during prometaphase in cell division,
- Collaborators: Scott Forth (biology), Meredith Betterton (UC Boulder), Joseph Klobusicky (U Scranton), John Fricks (Arizona State)
- linking the swimming and taxis properties to cells when they form colonies, particularly in choanoflagellates
- Collaborator: Yonatan Ashenafi (UC Alberta)
- inferring neuronal network topology from firing data via variational Bayesian methodology
- Collaborator: Gregor Kovačič
- Student: Devin Smith
- exploring hybrid dynamical modeling and neuronal network approaches to predicting biochemical changes in freshwater lakes under changing climactic environment
- Collaborator: Kevin Rose
- Students: Bertram Thomas
The understanding afforded by the first two basic science studies could have implications for synthetic nanotechnological devices intended to perform transport of materials.
Teaching
I generally teach classes involving probability and/or dynamics. I strive, when possible, to involve structured mathematical models in any class to show relevance of the material to real world applications, and to introduce another dimension of critical thinking into the pedagogy.
MATH 2400: Introduction to Differential Equations. I emphasize modeling and geometric understanding beyond the technical solution methods.
MATH 4300: Introduction to Complex Variables. This beautiful theory was very useful for clever approaches to problem solving, but this is now mostly buried in symbolic computing packages. So it should be viewed as a pure mathematics course, where the goal is to develop the capacity for abstract thought. As a single complex variable is two-dimensional, the theory can be largely connected with concrete visualization, until you realize that a complex function of a complex variable requires working in 4 real dimensions. Contemplating such functions, especially when conceptualized as Riemann surfaces, will exercise the imagination just beyond the limits of tangible realization in our universe.
MATH 4400: Ordinary Differential Equations and Dynamical Systems. There's not much useful in a purely technical sense more to learn than the material covered in MATH 2400, but we develop a geometric approach to viewing differential equations and their solutions which enables the understanding and analysis of differential equations without exact solutions.
MATH 4700: Foundations of Applied Mathematics. A summary of important elements of mathematics for problem solving in applications, introducing the "modeling'' approach and the various modes of reasoning involved beyond equation processing. I'm thinking about how to incorporate data science a bit into the course.
MATH 6590: Statistical Approaches to Differential Equation Models. This is a new course with the purpose of examining how data and statistical approaches can help characterize uncertainty in parameters or structure in a dynamical model. The first part of the class will review traditional statistical approaches, while the second part will examine some contemporary ideas on various forms of learning model structure from data.
MATH 6660: Stochastic Processes and Modeling. An introduction to probabilistic models that evolve in time. The focus is largely on discrete-time and continuous-time Markov chains, though we cover the basic methods that apply also to stochastic differential equations.
With Dr. Jeffrey Banks, I advise the mathematical science majors in the Class of 2026.
Publications
The following is a selection of recent publications in Scopus. Peter Kramer has 35 indexed publications in the subjects of Mathematics, Physics and Astronomy, Computer Science.