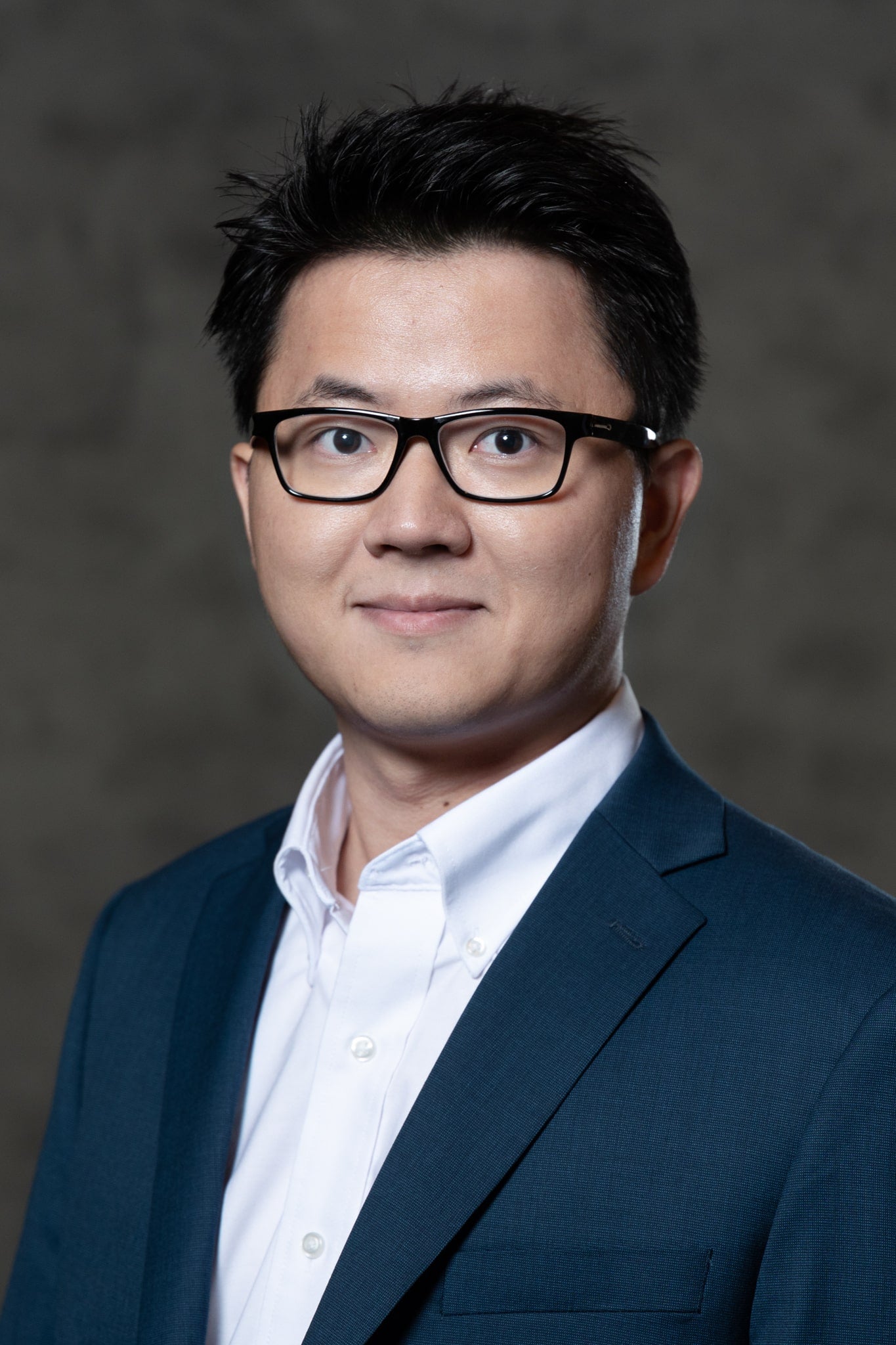
-
JEC 2032
- pans2@rpi.edu
-
518-276-6096
- 0000-0002-2462-362X
About
Shaowu Pan received his B.E. in Aerospace Engineering and B.S. in Applied Mathematics from Beihang University, China in 2013. After that, he received M.S. and Ph.D. in Aerospace Engineering and Scientific Computing from the University of Michigan, Ann Arbor in April 2021. Then he started as a Postdoctoral Scholar in the AI Institute in Dynamic Systems at the University of Washington, Seattle from 2021 to 2022. His research interests lie in the intersection between computational fluid dynamics, data-driven modeling of complex systems, scientific machine learning, and dynamical systems.
Ph.D., University of Michigan, 2021
M.S.E., University of Michigan, 2015
B.S. & B.E., Beihang University, 2013
Research
I'm interested in solving challenging modeling problems in large-scale complex nonlinear systems using theory of dynamical systems combined with tools from computational mathematics and deep learning.
Research interests
- AI for Science
- Physics-informed machine learning
- Data-Driven Control of High-dimensional Nonlinear Systems
- Differentiable Flow Physics
- Aerodynamics and Flow Control
- Turbulence Modeling
Ph.D. students
- Nithin Somasekharan
- Shahriar Akbar
- Weichao Li
Recognition
- MICDE Fellowship, University of Michigan, Ann Arbor
- Doctoral Fellowship, University of Michigan, Ann Arbor
- Rackham Summer Award, University of Michigan, Ann Arbor
- Richard and Eleanor Towner Prize for Outstanding Ph.D. Research (Department Nominee)
- Chinese Outstanding Student Abroad Award
- SIAM Student Travel Grant
Publications
- Pan, S., and K. Duraisamy. (2024). "On the Lifting and Reconstruction of Dynamical Systems with Multiple Attractors." Nonlinear Dynamics.
- Pan, S., Kaiser, E., Silva, B., Kutz, J., & Brunton, S. (2024). PyKoopman: A Python Package for Data-Driven Approximation of the Koopman Operator. Journal of Open Source Software.
- Yusuf, Soha, Jason E. Hicken, and Pan, S. (2024) "Constructing ILU preconditioners for advection-dominated problems using graph neural networks." AIAA AVIATION FORUM AND ASCEND.
- Pan, S., and Ozgur Tumuklu. (2024). "Composite Koopman Analysis of Hypersonic Nonequilibrium Flows Over a Double Wedge." AIAA AVIATION FORUM AND ASCEND.
- Ni, Y., Denolle, M. A., Shi, Q., Lipovsky, B. P., Pan, S., & Kutz, J. N. (2024). Wavefield reconstruction of distributed acoustic sensing: Lossy compression, wavefield separation, and edge computing. Journal of Geophysical Research: Machine Learning and Computation,
- Jang, B., A.A. Kaptanoglu, R. Gaur, S. Pan, M. Landreman, and W. Dorland. (2024). "Grad–Shafranov Equilibria via Data-Free Physics Informed Neural Networks." Physics of Plasmas 31, no. 3.
- Pan, S., S. Brunton, and N. Kutz. (2023). "Neural Implicit Flow: A Mesh-Agnostic Dimensionality Reduction Paradigm of Spatio-Temporal Data." Journal of Machine Learning Research.
- Pan, S., and E. Johnsen. (2017). "The Role of Bulk Viscosity on the Decay of Compressible, Homogeneous, Isotropic Turbulence." Journal of Fluid Mechanics.
- Pan, S., N. Arnold-Medabalimi, and K. Duraisamy. (2021). "Sparsity-Promoting Algorithms for the Discovery of Informative Koopman-Invariant Subspaces." Journal of Fluid Mechanics.
- Pan, S., and K. Duraisamy. (2018). "Data-Driven Discovery of Closure Models." SIAM Journal on Applied Dynamical Systems.
- Pan, S., and K. Duraisamy. (2020). "On the Structure of Time-Delay Embedding in Linear Models of Non-linear Dynamical Systems." Chaos: An Interdisciplinary Journal of Nonlinear Science.
- Pan, S., and K. Duraisamy. (2018). "Long-Time Predictive Modeling of Nonlinear Dynamical Systems Using Neural Networks." Complexity.
- Pan, S., and K. Duraisamy. (2020). "Physics-Informed Probabilistic Learning of Linear Embeddings of Nonlinear Dynamics with Guaranteed Stability." SIAM Journal on Applied Dynamical Systems.
- Singh, A.P., S. Pan, and K. Duraisamy. (2017). "Characterizing and Improving Predictive Accuracy in Shock-Turbulent Boundary Layer Interactions Using Data-Driven Models." 55th AIAA Aerospace Sciences Meeting.
- Bhatnagar, S., Y. Afshar, S. Pan, K. Duraisamy, and S. Kaushik. (2019). "Prediction of Aerodynamic Flow Fields Using Convolutional Neural Networks." Computational Mechanics.
- Sun, L., H. Gao, S. Pan, and J.-X. Wang. (2020). "Surrogate Modeling for Fluid Flows Based on Physics-Constrained Deep Learning Without Simulation Data." Computer Methods in Applied Mechanics and Engineering.
- Gao, Q., S. Pan, H. Wang, R. Wei, and J. Wang. (2021). "Particle Reconstruction of Volumetric Particle Image Velocimetry with the Strategy of Machine Learning." Advances in Aerodynamics.
- Ji, W., W. Qiu, Z. Shi, S. Pan, and S. Deng. 2021. "Stiff-PINN: Physics-Informed Neural Network for Stiff Chemical Kinetics." The Journal of Physical Chemistry.